-
Notifications
You must be signed in to change notification settings - Fork 10
New issue
Have a question about this project? Sign up for a free GitHub account to open an issue and contact its maintainers and the community.
By clicking “Sign up for GitHub”, you agree to our terms of service and privacy statement. We’ll occasionally send you account related emails.
Already on GitHub? Sign in to your account
gaussNorm is creating outliers (?) #33
Comments
We are not supporting gaussNorm. It's a legacy method developed over 10 years ago. You may want to look at using some of the more modern approaches published more recently.. |
Oh, that's a pity, I found it very useful... In addition to cytoNorm, what another normalization methods do you know, please? Thanks again. |
I agree, I think it is still useful for flow cytometry (and maybe mass also), especially when there are positive peak to ease anchoring at high range, because gaussNorm relies on the peak position rather than the identity of the distribution for quantile methods. |
Perfect, thanks a lot for your help! |
Are there any modern (or maintained) methods that don't require a control sample across batches? CytoNorm, batchadjust and FAUST all do. |
Dear RGLab team,
I'm running
gaussNorm
and it seems some outliers are being created... I've read #20 's answers but my data is correctly transformed.This is my first plot...
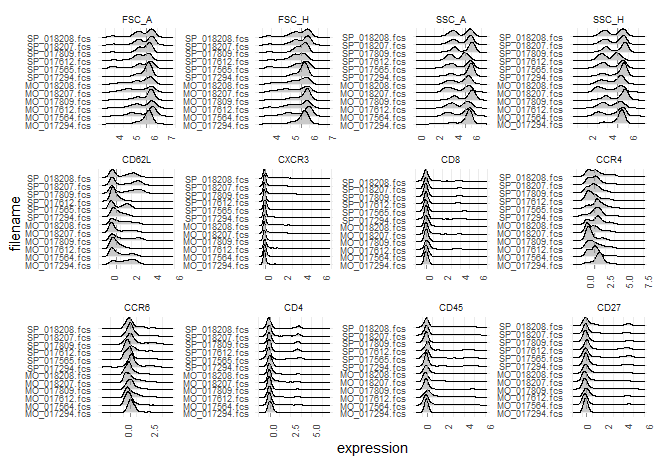
... and this another one is after normalization:
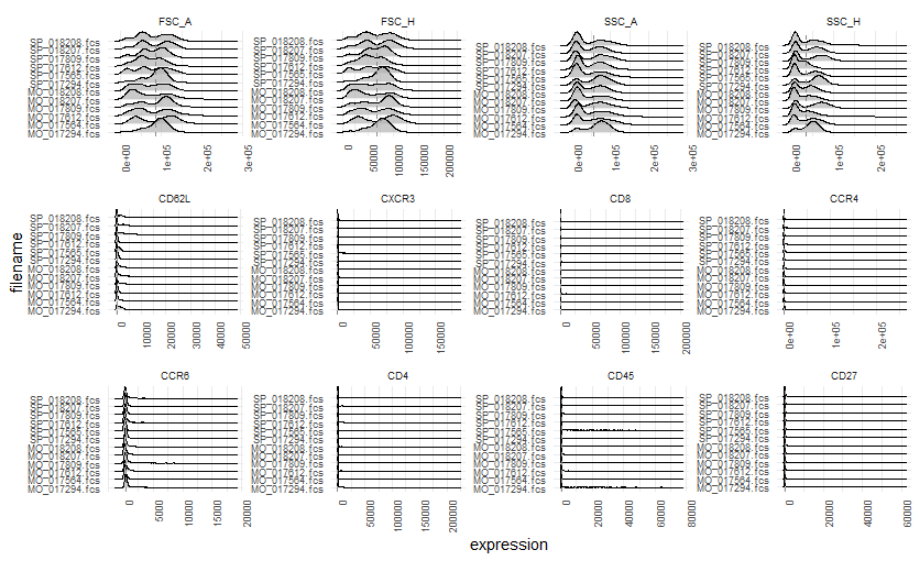
I guess that's because there're some outlier values....
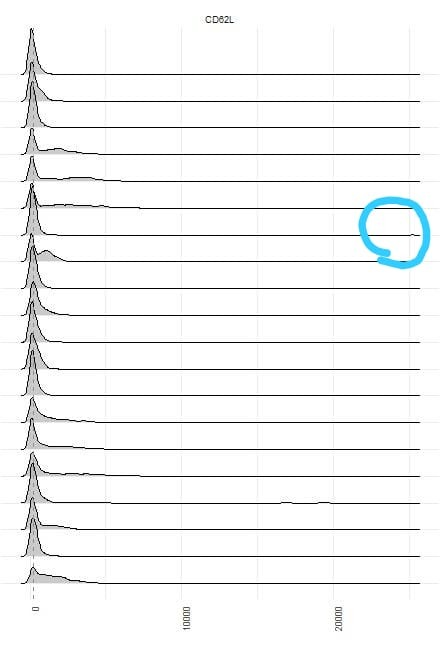
...but that aren't appearing in initial data:
Any idea, please, if I'm doing something wrong? Thanks in advance.
The text was updated successfully, but these errors were encountered: