You signed in with another tab or window. Reload to refresh your session.You signed out in another tab or window. Reload to refresh your session.You switched accounts on another tab or window. Reload to refresh your session.Dismiss alert
The evaluation function was not implemented in the code. So, I implemented it but I get a CUDA error which I traced back to transformers. I am hereby attaching the screenshot of the error. So for context I trained the relationNet using roberta as the encoder and then when I try to evaluate the trained model, I get this error.
The text was updated successfully, but these errors were encountered:
The evaluation function was not implemented in the code. So, I implemented it but I get a CUDA error which I traced back to transformers. I am hereby attaching the screenshot of the error. So for context I trained the relationNet using roberta as the encoder and then when I try to evaluate the trained model, I get this error.
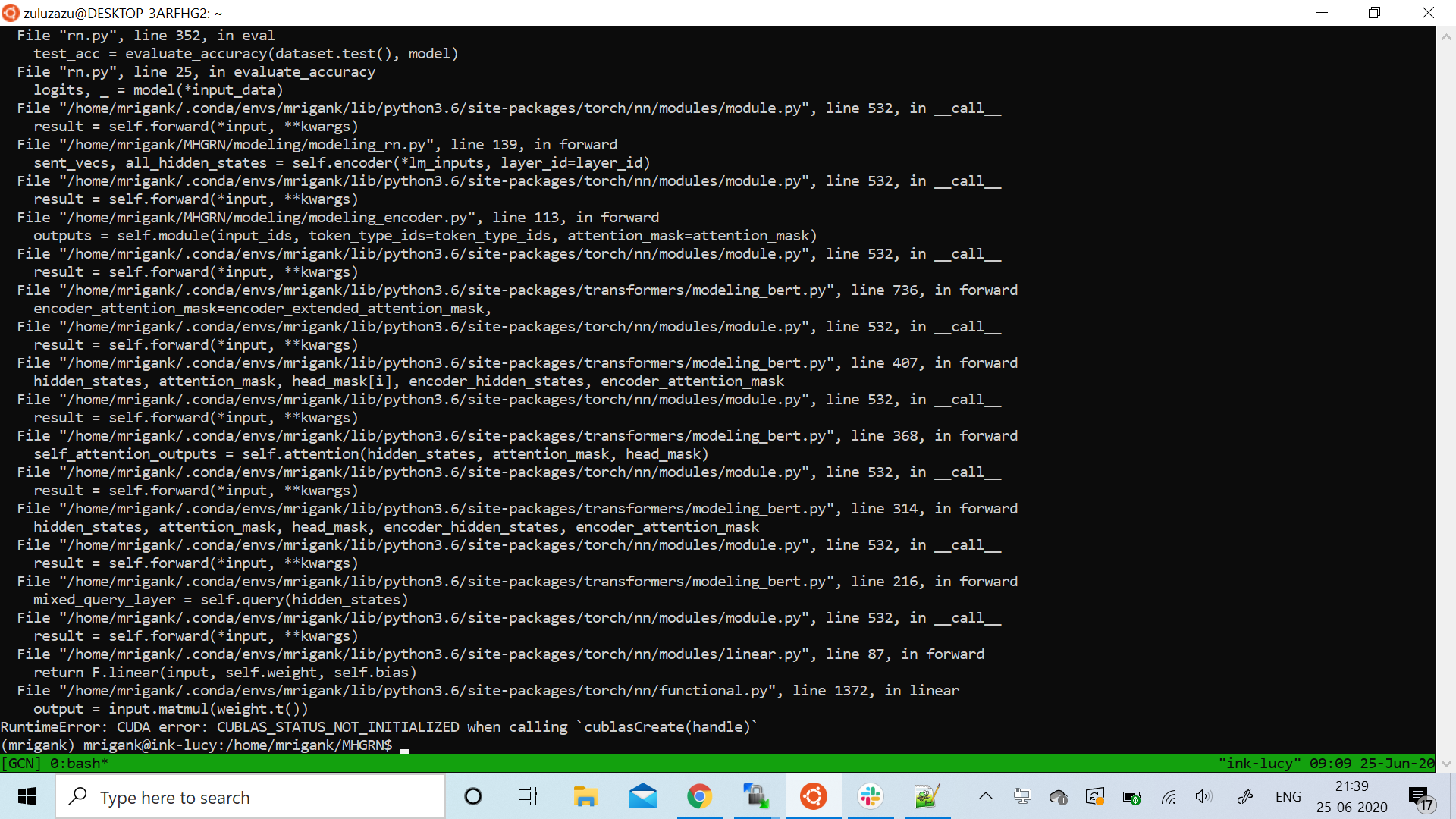
The text was updated successfully, but these errors were encountered: